
A Comprehensive Guide to Bayesian Statistics
Genre: eLearning | MP4 | Video: h264, 1280x720 | Audio: aac, 48000 Hz
Language: English | Size: 812 MB | Duration: 3h 13m
What you'll learn
An Overview on Statistical Inference
Frequentist vs Bayesian approach to Statistical Inference
Clearly understand Bayes Theorem and its application in Bayesian Statistics
Build a good intuitive understanding of Bayesian Statistics with real life illustrations
Master the key concepts of Prior and Posterior Distribution
Solve exam style numerical problems of computing Posterior Distribution for Population Parameter with different types of Prior
Understand Conjugate Prior and Jeffrey's Prior
Interval Estimation in Bayesian Statistics : Credible Intervals
Distinguish and work with Confidence Intervals and Credible Intervals
Solve problems of computing Credible Interval for Posterior Mean
Bayesian Hypothesis Testing: Bayes Factor
Learn to Interpret Bayes Factor
Solve numerical problems of computing Bayes Factor for two competing hypotheses
Build a solid understanding on Bayesian Decision Theory with examples
Decision Theory Terminology: State/Parameter Space, Decision Rule, Action Space, Loss Function
Minimizing Expected Loss
Real Life Illustrations of Bayesian Decision Theory
Use different Loss Functions: Squared Error Loss, Absolute Error Loss, 0-1 Loss
Decision Making with Frequentist vs Bayesian
Understand Bayesian Expected Loss, Frequentist Risk, and Bayes Risk
Admissibility of Decision Rules
Procedures to find Bayes Estimate & Bayes Risk: Normal & Extensive Form of Analysis
Solve numerical problems of computing Bayes Estimate and Bayes Risk for different Loss Functions
Bayesian's Defense & Critique
Applications of Bayesian Inference in various fields
Requirements
Basic knowledge of probability and statistics
You should be comfortable with concepts of conditional and marginal probability, all probability distributions, and basics of statistical inference
You will need concepts of differentiation and integration to solve the problems, so if you have that foundation, you'll be well prepared for this course.
To brush up the above concepts, a 'Prerequisite' document is provided in the first lecture of the course. Students are advised to go through it.
Description
This course is a comprehensive guide to Bayesian Statistics. It includes video explanations along with real life illustrations, examples, numerical problems, take away notes, practice exercise workbooks, quiz, and much more . The course covers the basic theory behind probabilistic and Bayesian modelling, and their applications to common problems in data science, business, and applied sciences.
The course is divided into the following sections:
Section 1 and 2: These two sections cover the concepts that are crucial to understand the basics of Bayesian Statistics-
An overview on Statistical Inference/Inferential Statistics
Introduction to Bayesian Probability
Frequentist/Classical Inference vs Bayesian Inference
Bayes Theorem and its application in Bayesian Statistics
Real Life Illustrations of Bayesian Statistics
Key concepts of Prior and Posterior Distribution
Types of Prior
Solved numerical problems addressing how to compute the posterior probability distribution for population parameters
Conjugate Prior
Jeffrey's Non-Informative Prior
Section 3: This section covers Interval Estimation in Bayesian Statistics:
Confidence Intervals in Frequentist Inference vs Credible Intervals in Bayesian Inference
Interpretation of Confidence Intervals & Credible Intervals
Computing Credible Interval for Posterior Mean
Section 4: This section covers Bayesian Hypothesis Testing:
Introduction to Bayes Factor
Interpretation of Bayes Factor
Solved Numerical problems to obtain Bayes factor for two competing hypotheses
Section 5: This section caters to Decision Theory in Bayesian Statistics:
Basics of Bayesian Decision Theory with examples
Decision Theory Terminology: State/Parameter Space, Action Space, Decision Rule. Loss Function
Real Life Illustrations of Bayesian Decision Theory
Classification Loss Matrix
Minimizing Expected Loss
Decision making with Frequentist vs Bayesian approach
Types of Loss Functions: Squared Error Loss, Absolute Error Loss, 0-1 Loss
Bayesian Expected Loss
Risk : Frequentist Risk/Risk Function, Bayes Estimate, and Bayes Risk
Admissibility of Decision Rules
Procedures to find Bayes Estimate & Bayes Risk: Normal & Extensive Form of Analysis
Solved numerical problems of computing Bayes Estimate and Bayes Risk for different Loss Functions
Section 6: This section includes:
Bayesian's Defense & Critique
Applications of Bayesian Statistics in various fields
Additional Resources
Bonus Lecture and a Quiz
At the end of the course, you will have a complete understanding of Bayesian concepts from scratch. You will know how to effectively use Bayesian approach and think probabilistically. Enrolling in this course will make it easier for you to score well in your exams or apply Bayesian approach elsewhere.
Complete this course, master the principles, and join the queue of top Statistics students all around the world.
Who this course is for:
Students currently pursuing Statistics and Probability
Anyone who wants to build a strong fundamental of Bayesian Statistics
Anyone who wants to apply Bayesian Statistics to other fields like ML, Artificial Intelligence, Business, Applied Sciences, Psychology etc.
Students of Machine Learning and Data Science
Data Scientists curious about Bayesian Statistics
https://rapidgator.net/file/919693fdf95642f46889eb75f8981682/bayesian_statistics_w.rar.html
http://alfafile.net/file/8rLuJ
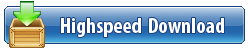